Publication
Article
The American Journal of Managed Care
Primary Care Capacity as Insurance Coverage Expands: Examining the Role of Health Information Technology
Author(s):
Primary care physicians using more health information technology were less likely to accept new patients.
Objectives
Under the Affordable Care Act, many newly insured Americans have the challenge of establishing care with a primary care physician (PCP). We sought to examine whether health information technology (HIT) use in primary care practices was associated with anticipated capacity to accept new patients.
Study Design
Secondary analysis of a cross-sectional survey of Michigan PCPs from the specialties of pediatrics, internal medicine, and family medicine, conducted from October to December 2012. HIT use was considered independently for 8 types of HIT and in aggregate as a total count of HIT in use. Primary care capacity was assessed as self-reported capacity to accept new patients.
Results
Of 739 respondents, 83% reported they anticipated capacity to accept new patients. In multivariable analysis, we found that physicians using a greater number of HITs were significantly less likely to anticipate capacity to accept new patients (adjusted odds ratio [OR] = 0.86; 95% CI, 0.76-0.97). PCPs with higher HIT use were also less likely to accept patients with private insurance (adjusted OR 0.87; 95% CI, 0.77-0.97), but not with Medicaid (adjusted
OR 0.94; 95% CI, 0.84-1.05) or Medicare (adjusted OR 0.91; 95% CI, 0.83-1.01). Among individual HITs, electronic health records (adjusted OR 0.54; 95% CI, 0.30-0.96) and electronic access to admitting hospital records (adjusted OR 0.46; 95% CI, 0.22-0.96) were the only HITs significantly associated with lower anticipated primary care capacity.
Conclusions
PCPs using a greater number of HITs were less likely to anticipate capacity to accept new patients. Implementation of HIT and other practice innovations must be carefully coordinated to optimize capacity to care for the newly insured.
Am J Manag Care. 2014;20(11 Spec No. 17):SP547-SP554
Health information technology (HIT) has been widely touted for its potential to improve primary care practice efficiency and capacity, but this study’s findings call into question whether this is occurring.
- Physicians using a greater number of HITs were significantly less likely to anticipate capacity to accept new patients.
- In an era of concurrent HIT and insurance coverage expansions, policy makers must weigh the unintended consequences of each in order to optimize capacity to care for the newly insured.
Millions of Americans who have recently gained insurance coverage through the Patient Protection and Affordable Care Act (ACA) now face the challenge of establishing care with a primary care pro
vider (PCP). For coverage to translate to access, primary
care providers will need adequate capacity to accept new
patients. Efforts have long been under way to increase
primary care capacity, with a principal emphasis on ex
panding the primary care workforce.1 More recently, innovations in primary care practices, such as improvements in clinic work flow and care coordination, have also been in- troduced to improve both capacity and efficiency of care.
2,3 Many of these innovation efforts have included the implementation of health information technology (HIT), such as electronic health records (EHRs), patient portals, and reminder systems.
Since the passage of the Health Information Technology for Economic and Clinical Health (HITECH) Act of 2009, HIT has been widely promoted as potentially cost- and time-saving for practices, and the early stages of “meaningful use” of these technologies have been heavily incentivized. While some studies have shown improvements in practice efficiency associated with use of EHR and other HIT resources,4-8
others have shown decreases in efficiency or mixed results.
9-20 It is conceivable that improvements in efficiency could allow primary care physicians to see more patients in the office, thus increasing their overall capacity. In fact, this very idea has been espoused by the Office of the National Coordinator for Health Information Technology.21 Nonetheless, empirical data to support the connection between HIT use and primary care capacity are limited, and it is possible that adjusting to newly implemented HITs may instead cause physicians to limit their efficiency and have reduced capacity to see patients. Our objective was to examine whether use of HIT in primary care practices was independently associated with anticipated capacity to accept new patients.
METHODS
Study Design
We performed a secondary analysis of data from the Center for Healthcare Research & Transformation and the University of Michigan Child Health Evaluation and Research Unit 2012 Survey of Michigan Physicians. The purpose of this cross-sectional survey was to understand the challenges PCPs face in their practices during the era of health reform, with a focus on anticipated capacity to care for newly insured residents. Questions were developed by members of the research team experienced in survey design (MR, MUP, MMD). Survey questions were piloted with primary care physicians and modified to optimize respondent understanding.
Between October and December 2012, surveys were mailed to PCPs across the state of Michigan. Potential participants received up to 3 mailings, and a $5 incentive was included in the first mailing to encourage response. The study was granted exempt status by the University of Michigan Medical School Institutional Review Board, as it included only de-identified data.
Sample
Five hundred Michigan physicians from each of 3 primary care specialties (pediatrics, internal medicine, and family medicine) were randomly selected from the American Medical Association (AMA) Physician Masterfile, a comprehensive list that includes both AMA members and nonmembers, for a total of 1500 physicians in the original sample. Physicians who responded but reported they were not practicing primary care (eg, retired, or exclusively practicing inpatient medicine) were excluded from the analysis.
Measures
Primary Care Capacity.
The primary outcome was PCPs’ assessment of their future capacity to accept new patients. Participants were asked, “If the number of Michigan patients with insurance coverage increases in the future, will you have the capacity to accept additional
patients?” with yes/no response options (see
secondary outcome.
eAppendix A, available at www.ajmc.com) for all relevant questions from the survey instrument. For those who responded yes, we also asked about ability to expand practice capacity by payer type (eg, for patients with private insurance, Medicaid, or Medicare) and examined this as a
HIT use.
The primary predictor variable was PCPs’
use of HIT. Participants were asked whether they utilized any of 8 types of HIT (see eAppendix A): EHRs, patient registries, electronic prescribing, Web portals for patients to schedule their own appointments, Web portals for patients to request prescription refills, reminder/ recall systems to contact patients about recommended services, state immunization registry participation, and electronic access to admitting hospital records. A panel of stakeholders from the Michigan State Medical Society and the Michigan Osteopathic Association was convened to develop survey items that corresponded to meaningful use and patient-centered medical home initiatives that primary care physicians faced at the time of the survey. After consensus-building among panel participants, the HIT types that best represented current incentives for implementation in primary care practices were selected for inclusion in the survey. We examined each HIT type independently, and also in aggregate by creating an index of HIT use. The index was defined as a total count of the number of HITs currently in use by the physician.
Survey items included physician demographic characteristics such as gender, specialty, number of years in practice (<10 years, 10-20 years, or >20 years, categorized for the multivariable analysis as either ≤20 or >20 years), and self-reported weekly visit volume (categorized as ≤100 or >100 patients seen per week per physician), as well as practice characteristics such as size (number of physicians in practice considered as a continuous variable), self-reported current payer mix, and zip code of the practice.
Covariates.
For payer mix, we created a composite variable to determine the predominant payer for the practice. A payer (eg, private insurance, Medicaid, or Medicare) was considered predominant if a physician had only 1 payer type that constituted more than 30% of the physician’s patient population. We defined a “mixed” payer category for those practices with more than 1 payer representing greater than 30% of patients, or with no predominant payer representing at least 30% of patients. We assessed urbanicity of the practice setting by linking Federal Information Processing Standard county codes obtained from self-reported zip codes to the US Department of Agriculture Economic Research Service 2013 Urban Influence Codes. Urban Influence Codes provide a standard 12-point classification scheme to distinguish counties by population density and proximity of the population to the largest town or city, allowing categorization into metropolitan (Urban Influence Codes 1-2) and nonmetropolitan (Urban Influence Codes 3-12) counties.22
Statistical Analysis
We used standard descriptive statistics to characterize physician respondents’ baseline characteristics, as well as their overall use of HIT and anticipated capacity to accept new patients. We then used logistic regression to perform bivariate analyses of associations between predictor variables and the primary outcome of capacity, as well as the secondary outcome of capacity by payer type. To examine the independent relationship between HIT use and primary care capacity after adjusting for covariates, we conducted multivariable logistic regression analysis and expressed the results as adjusted odds ratios (ORs) with 95% confidence intervals. Using the same estimation model, we also obtained the adjusted predicted probabil- ity of capacity to accept new patients at different numbers of HIT in use. A 2-sided P <.05 was considered statistically significant. We included the physician-, practice- and community-level covariates mentioned above in order to control for potential confounders. The unit of analysis was the physician.
In order to examine possible moderation of the HIT/capacity relationship by practice size, we subsequently included an interaction term between HIT use and practice size in the logistic regression model. We assessed the main relationship within each group (eg, within small practice size) and compared that between groups (small vs large practice size).
The proportion of responses with missing data was less than 5% for all individual items. To address missing values in the final multivariable model (12% in aggregate), we performed multiple imputation by using a chained equation23,24 and generated 10 replications of the imputed data set. We repeated our main multivariable analysis with the imputed data sets and observed similar results to the analysis using the non-imputed data set (ie, with no significant difference in effect size or confidence intervals). The results reported here are from the original, non-imputed dataset.
To assess goodness of fit, we checked the final model with both the area under the curve method (C statistic = 0.69) and the Hosmer-Lemeshow goodness of fit test (which was nonsignificant, suggesting good model fit). All analyses were performed using STATA version 13 (Stata Corp, College Station, Texas).
RESULTS
Survey respondents (N = 739, response rate = 49%) were similar to the original sample of physicians from the AMA Physician Masterfile (see eAppendix B
). While the response rate is lower than in surveys of nonphysicians, it is consistent with other surveys of physicians with small financial incentives. The respondents included an approximately equal number of men and women (356 women, or 48%), with similar representation from each of the 3 specialties (
Table 1). Most physician respondents had been in practice for more than 10 years (76%) and saw fewer than 100 patients in a typical week (66%). Sixty-one percent of physician respondents practiced in settings with fewer than 6 physicians. Many practices accepted a diversity of payer types, with 43% of practices having no predominant payer. The majority of respondents’ practices (85%) were located in urban areas.
In aggregate, PCPs used a mean of 5.1 HITs (SD = 1.9) in their practices. Among these, the most common HIT in use was electronic prescribing (89% of PCPs). The least common HIT in use was a Web portal for patients to schedule their own appointments (21% of PCPs) (Figure 1).
Overall, 83% of PCPs reported they would have capacity to accept new patients in the future. In bivariate analyses, with each incremental increase in the number of HITs in use, there were significantly lower odds of self-reported primary care capacity (OR = 0.87; 95% CI, 0.78-0.97).
In multivariable analysis adjusting for physician-, practice- and community-level covariates, the odds of self-reported primary care capacity decreased by 14% with each additional HIT in use (adjusted OR = 0.86; 95% CI, 0.76- 0.97) (ORs reported in Table 2
and predicted probabilities of capacity presented in
Figure 2). Among other covariates, greater number of years in practice was significantly associated with lower odds of capacity. Physicians who specialized in pediatrics, had a high visit volume, or had a predominantly Medicare payer mix had significantly greater odds of capacity.
For the secondary outcome of capacity by payer type among respondents who reported they had capacity to accept new patients, we found that greater use of HITs was associated with significantly lower odds of anticipated capacity to accept privately insured patients (adjusted OR 0.87; 95% CI, 0.77-0.97) (Table 2). However, use of HIT was not significantly associated with lower odds of anticipated capacity to accept patients with either Medicaid (ad
justed OR 0.94; 95% CI, 0.84-1.05) or Medicare (adjusted OR 0.91; 95% CI, 0.83-1.01).
Table 3
Among individual HIT types examined, electronic health records (adjusted OR 0.54; 95% CI, 0.30-0.96) and electronic access to admitting hospital records (adjusted OR 0.46; 95% CI, 0.22-0.96) were each significantly associated with lower odds of self-reported capacity (). All other HIT types examined (electronic prescribing, state immunization registry, patient registry, reminder/ recall system, and Web portals to either request refills or schedule appointments) were not significantly associated with anticipated capacity.
We also examined possible variation in the association between HIT use and capacity by practice size. We found that in smaller practices (1 to 5 physicians), the inverse relationship remained significant (adjusted OR 0.83; 95% CI, 0.71-0.97), while in larger practices (>5 physicians) the association was not present (adjusted OR 0.95; 95% CI, 0.79-1.15).
DISCUSSION
In this cross-sectional study of a representative sample of Michigan primary care physicians, we found that physicians using a greater number of health information technologies were significantly less likely to anticipate capacity to accept new patients. PCPs with higher HIT use were also specifically less likely to accept patients with private insurance, but not with Medicaid or Medicare. Among individual HITs examined, electronic health records and electronic access to admitting hospital records were significantly associated with lower anticipated primary care capacity. Additionally, we found that the inverse association of HIT use and capacity may be more apparent in small versus large practices.
10,12,18
12
4-8
6
7
Our findings have a few possible interpretations. First, it is plausible that use of technologies may lead to decreased physician efficiency, and that decreased efficiency leads to decreased capacity to see additional patients. Several prior studies have found either increased time per patient encounter or decreased physician or practice productivity associated with HIT use.One small study also showed downstream negative impact on patients’ ability to access care.However, other studies,including one review of the literature,have instead found improved physician productivity with HIT use. In one study of 42 primary care practices across the United States between 2006 and 2009, while there was improved physician productivity associated with EHR use in large practices, there was decreased productivity in small practices, suggesting this association may depend on practice size or other characteristics.Our results suggest a similar practice-size-related phenomenon in this statewide sample of primary care physicians. Furthermore, previous studies have defined “efficiency” or “productivity” in myriad ways—including as time spent per patient encounter, work relative value units, overall “work burden” of physicians, care utilization, practice revenue, and patient visit volume—which may partly explain the mixed results in the literature.
13,20
Second, it is also possible that any potential decline in efficiency may be only temporarily associated with the HIT implementation period, as physicians take time to get accustomed to a new technology. A few studies have found that this implementation-related reduction in efficiency may last up to 12 months.Because the timing of our survey in late 2012 corresponded to the end of the 2011-2012 rollout of meaningful use stage 1 incentives for EHR implementation, it is conceivable that our findings represent only a temporary implementation period effect. However, this timing of EHR incentive rollout and possible implementation period drop in productivity may not necessarily explain the additional inverse association we found between anticipated primary care capacity and other types of HIT, such as having access to admitting hospital records.
25
Third, it could be that practices with higher HIT use are generally well resourced and that these types of practices typically see more affluent patients. Such practices may have reservations about accepting newly insured patients, who are often of low socioeconomic status and may represent a different demographic than that typically seen in those practices.In this way, HIT use may be a marker of well-resourced practices. However, we controlled for predominant payer mix, a variable related to overall practice resources, and continued to find a significant inverse association between HIT use and anticipated capacity.
In addition, it was surprising to find that greater HIT use was associated specifically with lower anticipated capacity for privately insured patients, but not with Medicaid or Medicare patients, particularly since private insurance frequently provides higher reimbursements than Medicaid or Medicare. We speculate about 2 possible explanations for this finding. First, with the increase of Medicaid reimbursement rates for primary care physicians to the level of Medicare reimbursement rates during this period, new patients with Medicaid or Medicare may have appeared more attractive due to a steadier and predictably high level of reimbursement. Second, as noted above, it is possible that practices with the resources to adopt HIT and with a typically higher proportion of privately insured patients may be less likely to accept new patients generally.
26
This study should be interpreted in the context of several potential limitations. First, our cross-sectional data limits inferences regarding causal relationships. We did not characterize the timeline of HIT implementation in our study, and it is possible practice efficiency and capacity could improve after HIT implementation. Nevertheless, given the national timeline of EHR meaningful use incentives noted above, it is likely that our findings represent the association of capacity with the first 1 to 2 years following HIT implementation. Second, we did not distinguish which HIT vendors were used by physicians, and given differences in user interface, different brands may have varying impacts on practice capacity. Third, it is possible that the variables of physician specialty and predominant payer mix were collinear (eg, internists are more likely to accept Medicare patients, and pediatricians are more likely to accept Medicaid patients), but there was no evidence of multicollinearity to suggest that our estimates of the HIT/capacity association were biased. Fourth, we relied on respondents’ self-reported HIT use and likelihood of accepting new patients. While there is a potential for social desirability bias in both types of measures, surveys are the commonly used method for assessing these aspects of practice.Fifth, we did have missing data in our multivariable model, but this was unlikely to have impacted the findings since our sensitivity analyses using multiple imputations demonstrated equivalent results. Furthermore, we considered conducting the analysis with hierarchical regression, but had insufficient objective data at the practice and community levels to inform the model. Therefore, we fit the model assigning practice-level and community-level characteristics as reported by each physician. Lastly, while our results are representative of primary care physicians in a large Midwestern state, they may not be generalizable to all US states.
21
The notion that HIT expansion necessarily translates into improved efficiency and capacity in primary care practices has been widely disseminated.Our findings call into question whether this is occurring, at least during this early implementation time period. In an era of concurrent expansion of health information technology through the HITECH Act and expansion of insurance coverage through the ACA, policy makers must weigh the unintended consequences of each in order to maximize improvements in both healthcare quality and access. This is a challenging undertaking, and one best informed by data-driven approaches. Further research, such as additional physician surveys or simulated patient studies examining actual acceptance of new patients, is needed to better understand the impact of HIT implementation on access to primary care for newly insured individuals over time.
Acknowledgments
The authors thank Lauren S. Hughes, MD, MPH, and Michelle H. Moniz, MD, both of the Robert Wood Johnson Foundation Clinical Scholars Program at the University of Michigan, for thoughtful comments on drafts of this article. The authors also thank Elyse N. Reamer, BS, of the University of Michigan Medical School, for assistance with literature review, as well as Jessica Landgraf, MA, of the of the Robert Wood Johnson Foundation Clinical Scholars Program at the University of Michigan, and Laura Spera, MS, MCS, of the University of Michigan Child Health Evaluation and Research Unit, Division of General Pediatrics, for assistance with data management.
Author Affiliations: Robert Wood Johnson Foundation Clinical Scholars Program, University of Michigan, Ann Arbor (RT, HJC, MMD); Division of General Medicine, Department of Internal Medi- cine, University of Michigan, Ann Arbor (RT, HJC, MMD); Institute for Healthcare Policy and Innovation, University of Michigan, Ann Arbor (RT, MMD); Center for Healthcare Research & Transformation, Ann Arbor, MI (EN, MR, DY, MU-P); City of Detroit Department of Health and Wellness Promotion, Detroit, MI (RR); Child Health Evaluation and Research Unit, Department of Pediatrics, University of Michigan, Ann Arbor (RR, MMD).
Source of Funding: Center for Healthcare Research & Transforma- tion; Robert Wood Johnson Foundation Clinical Scholars Program.
Author Disclosures: Dr Davis serves as chief medical executive for the State of Michigan. The findings described herein are those of the authors and do not necessarily reflect the views of the State of Michigan.
Authorship Information: Concept and design (RT, EGN, MR, DY, MUP, MMD); acquisition of data (MR, RR, MU-P, MMD); analysis and interpretation of data (RT, EGN, MR, HC); drafting of the manuscript (RT, EGN); critical revision of the manuscript for important intellectual content (RT, EGN, MR, HC, RR, DY, MU-P, MMD); statistical analysis (RT, HC); obtaining funding (MU-P); administrative, technical, or logistic support (EGN, MR, RR, DY); and supervision (RT, MMD).
Address correspondence to: Renuka Tipirneni, MD, MSc, Robert Wood Johnson Foundation Clinical Scholar, Clinical Lecturer in Inter- nal Medicine, University of Michigan, 2800 Plymouth Rd, Bldg 10, Rm G016, Ann Arbor, MI 48109. E-mail: rtipirne@med.umich.edu.
1. Colwill JM, Cultice JM, Kruse RL. Will generalist physician supply meet demands of an increasing and aging population?
. 2008;27(3):w232-w241.
2. BodenheimerTS, Smith MD. Primary care: proposed solutions to the physician shortage without training more physicians.
Health Aff (Millwood). 2013;32(11):1881-1886.
Health Aff (Millwood)
3. Shipman SA, Sinsky CA. Expanding primary care capacity by reducing waste and improving the efficiency of care.
. 2013;32(11):1990-1997.
4. Joos D, Chen Q, Jirjis J, Johnson KB. An electronic medical record in primary care: impact on satisfaction, work efficiency and clinic processes.
AMIA Annu Symp Proc. 2006:394-398.
Health Aff (Millwood)
5. Cheriff AD, Kapur AG, Qiu M, Cole CL. Physician productivity and the ambulatory EHR in a large academic multi-specialty physician group.
. 2010;79(7):492-500.
6. Fontaine P, Ross SE, ZinkT, Schilling LM. Systematic review of health information exchange in primary care practices.
. 2010;23(5):655-670.
Int J Med InformJ Am Board Fam Med
7. Adler-Milstein J, Huckman RS.The impact of electronic health record use on physician productivity.
. 2013;19(10 Spec No 1):SP345-SP352.
8. Weiner JP, Yeh S, Blumenthal D. The impact of health information technology and e-health on the future demand for physician services.
. 2013;32(11):1998-2004.
Am J Manag CareHealth Aff (Millwood)
9. Pizziferri L, Kittler AF, Volk LA, et al. Primary care physician time utili- zation before and after implementation of an electronic health record: a time-motion study. J Biomed Inform
. 2005;38(3):176-188.
10. Poissant L, Pereira J,Tamblyn R, KawasumiY.The impact of elec- tronic health records on time efficiency of physicians and nurses: a systematic review.
. 2005;12(5):505-516.
J Am Med Inform Assoc
11. Chaudhry B, Wang J, Wu S, et al. Systematic review: impact of health information technology on quality, efficiency, and costs of medical care.
. 2006;144(10):742-752.
12. Samaan ZM, Klein MD, Mansour ME, DeWittTG.The impact of the electronic health record on an academic pediatric primary care center.
J Ambul Care Manage
. 2009;32(3):180-187.
Ann Intern Med13. De Leon S, Connelly-Flores A, Mostashari F, Shih SC. The business end of health information technology. can a fully integrated electronic health record increase provider productivity in a large community practice?
. 2010;25(6):342-349.
J Med Pract Manage
14. Furukawa MF. Electronic medical records and efficiency and pro- ductivity during office visits.
. 2011;17(4):296-303.
Am J Manag Care
15. Goetz Goldberg D, Kuzel AJ, Feng LB, DeShazo JP, Love LE. EHRs in primary care practices: benefits, challenges, and successful strategies.
. 2012;18(2):e48-e54.
Am J Manag Care
16. Howard J, Clark EC, Friedman A, et al. Electronic health record im- pact on work burden in small, unaffiliated, community-based primary care practices.
. 2013;28(1):107-113.
J Gen Intern Med
17. BishopTF, Press MJ, Mendelsohn JL, Casalino LP. Electronic com- munication improves access, but barriers to its widespread adoption remain.
. 2013;32(8):1361-1367.
Health Aff (Millwood)
18. ZhouY, Ancker JS, Upadhye M, et al.The impact of interoperabil- ity of electronic health records on ambulatory physician practices: a discrete-event simulation study.
. 2013;21(1):21-29.
Inform Prim Care
19. Goldzweig CL, Orshansky G, Paige NM, et al. Electronic patient portals: evidence on health outcomes, satisfaction, efficiency, and attitudes: a systematic review.
. 2013;159(10):677-687.
Ann Intern Med
20. Fleming NS, Becker ER, Culler SD, et al.The impact of electronic health records on workflow and financial measures in primary care practices.
. 2014;49(1, pt 2):405-420.
Health Serv Res
21. Buntin MB, Jain SH, Blumenthal D. Health information technol- ogy: laying the infrastructure for national health reform.
. 2010;29(6):1214-1219.
Health Aff (Millwood)
22. United States Department of Agriculture (USDA), Economic Re- search Service. Urban Influence Codes. USDA website. http://www .ers.usda.gov/data-products/urban-influence-codes/documentation.aspx#.UzsY9z1dWGc. Published 2013. Accessed May 15, 2014.
23. Royston P. Multiple imputation of missing values: further up- date of with an emphasis on categorical variables.
. 2009;9(3):466-477.
Stata Journal
24. White IR, Royston P, Wood AM. Multiple imputation using chained equations: issues and guidance for practice.
. 2011;30(4):377-399.
Stat Med
25. Chirayath HT. Who serves the underserved? predictors of physician care to medically indigent patients.
. 2006;10(3):259-282.
Health (London)
26. Jha AK, Ferris TG, Donelan K, et al. How common are electronic health records in the United States? a summary of the evidence. Health Aff (Millwood). 2006;25(6):w496-w507.
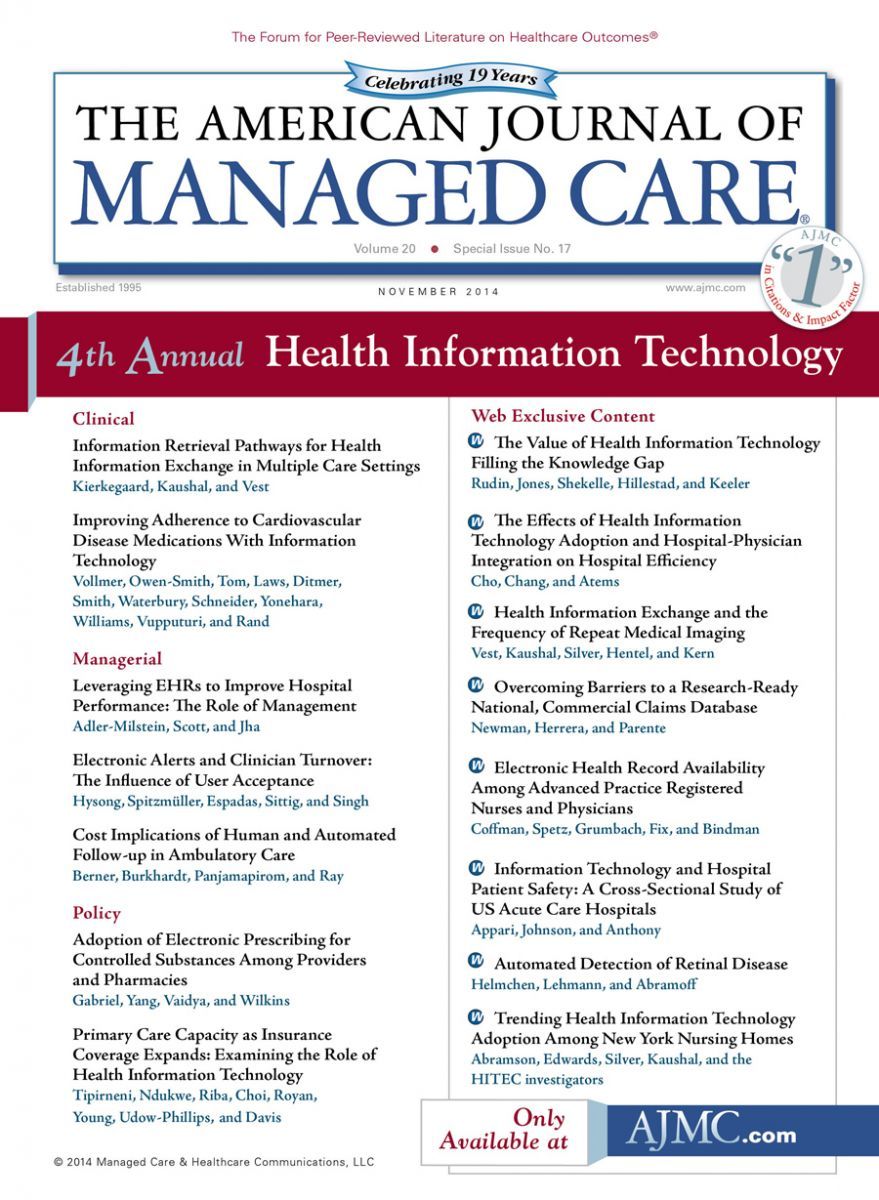
Newsletter
Stay ahead of policy, cost, and value—subscribe to AJMC for expert insights at the intersection of clinical care and health economics.