Publication
Article
The American Journal of Managed Care
Primary Care Diabetes Bundle Management: 3-Year Outcomes for Microvascular and Macrovascular Events
Author(s):
Using a system for primary care management of patients with diabetes may reduce the risk of myocardial infarction, stroke, and retinopathy over a 3-year period.
Objectives
To determine whether a system of care with an all-or-none bundled measure for primary-care management of diabetes mellitus reduced the risk of microvascular and macrovascular complications compared with usual care.
Study Design
A parallel pre-post observational design was used. In 2006, a system of care for diabetes was implemented for some members of the Geisinger Health Plan. A total of 4095 primary-care patients were in the Diabetes System of Care group (DS) and compared with a propensity score—matched cohort of 4095 primary care patients not in the system of care (non-Diabetes System of Care [NDS]).
Methods
Cumulative hazard rate was measured over a 3-year period for retinopathy, amputation, stroke, and myocardial infarction (MI).
Results
The adjusted hazard ratios (HRs) for MI (HR, 0.77; 95% CI, 0.65- 0.90), stroke (HR, 0.79; CI, 0.65-0.97), and retinopathy (HR, 0.81; CI, 0.68-0.97) were all significantly lower among DS patients. The adjusted HR for major amputations (HR, 1.32; CI, 0.45-3.85) did not differ between groups, but only 17 major amputations occurred during the follow-up period. The necessary number of patients to treat in order to prevent 1 event over 3 years was 82 for MI, 178 for stroke, and 151 for retinopathy.
Conclusions
A system of care with an all-or-none bundled measure used in primary care for patients with diabetes may reduce the risk of MI, stroke, and retinopathy over a 3-year period.
Am J Manag Care. 2014;20(6):e175-e182
A diabetes system of care using an all-or-none bundled measure can reduce the microvascular and macrovascular complications of diabetes in only 3 years.
- A system of care for diabetes can reduce myocardial infarction, stroke, and retinopathy in only 3 years.
- Translation into a workable care protocol in primary care is challenging. Workflows were developed that were measurable, scalable, reliable, and not dependent on the diligence of individual providers.
- Payers and health systems can innovate and invest together in the infrastructure to build similar systems of care, knowing that the benefit for their patients with diabetes will be prompt.
The prevalence of diabetes mellitus in the United States increased 40% between 2007 and 2010, to 11.9% of the population aged 20 years and older.1 Patients with diabetes are at increased risk for microvascular complications including retinopathy, nephropathy and amputation, and macrovascular complications including stroke and myocardial infarction (MI). Glycemic control has dramatically improved microvascular outcomes in patients with type 1 diabetes2 and type 2 diabetes,3 but has not consistently been associated with improved macrovascular outcomes, such as cardiovascular disease (CVD). Recent studies show that intensive glucose control is associated with increased risk or no benefit.4-6 In contrast, lipid and blood pressure (BP) control have resulted in clear reductions in CVD in persons with diabetes.7-9 Moreover, the combined control of these risk factors reduced the risk of CVD outcomes.10,11 Notably, the time to onset of risk reduction can be relatively short. For example, smoking cessation reduces coronary events within 6 months for patients at high risk.12
In spite of the evidence, translation of standards of care into a workable care protocol in primary care is challenging. The American Diabetes Association (ADA) has promulgated detailed standards of care for improving patient outcomes, including recommendations for medical care from physician-coordinated teams.13 However, providing state-of-the- art guideline care is rarely achieved.14 Although there is evidence that the use of an electronic health record (EHR) may improve process-of-care measures and intermediate outcome measures for diabetes15 even when guideline care is provided, it is unclear whether the benefits of reduced risk accrue and how much time is required before risk reduction is measurable.
A team-based model was designed to more consistently provide evidence-based care to patients with diabetes. This system of care used a 9-component bundle of diabetes measures, a workflow redesign, EHR tools, and financial incentives to improve the consistency and reliability of care, as previously reported.16 A key aspect of this program is an all-or-none measure that determines physician incentives. We describe the impact of this system of care on risk of microvascular complications of retinopathy and amputation and macrovascular complications of stroke and MI over the first 3 years of implementation.
METHODS
This research study was approved by the Geisinger Institutional Review Board. The evaluation of the diabetes system of care was completed among members of the Geisinger Health Plan (GHP). Approximately 45% of GHP members receive their care from Geisinger primary care physicians. GHP also contracts with non-Geisinger physicians who provide primary care to the other 55% of members. The diabetes system of care was implemented in all 38 Geisinger primary care sites, referred to hereafter as Diabetes System (DS). DS patients were compared with propensity score—matched primary care patients from other clinics in the same region, referred to hereafter as non-Diabetes System (NDS). One key part of the DS is that it was implemented in an integrated healthcare system. This allowed for many advantages in implementation, such as a shared EHR system across all providers, ease of reporting to all providers without conflict, and an equitable application of physician incentives to all providers. The NDS system had many nonintegrated care characteristics, yet had numerous diabetes improvement programs in effect during the study period that are discussed further in the discussion section.
Diabetes System of Care (DS)
Table 1
The DS was designed by a multispecialty, multidisciplinary work group. The ADA Guidelines for the Standard of Medical Care for Diabetes were used as the basis for the system of care.17 The team model included an allor- none measure with physician incentives awarded only when a patient fulfilled all 9 components of the bundle (). Previous research has examined compliance rates within the DS program and found significant increases in all measures of diabetes care within the first 12 months.18
Beginning in January 2006, the all-or-none bundle was routinely measured in all adults with diabetes at Geisinger primary care sites. DS included delegated accountable responsibilities for each team member—physician, advanced practitioner, nurse, case manager, front office staff, and call center staff. Workflows were developed to be measurable, scalable, reliable, and not dependent on the diligence of individual providers. DS included EHR decision support, autogenerated patient report cards, and automated updating of a patient registry. During the office visit, licensed practical nurses and medical assistants were presented with decision support for all process-related diabetes care and had standing orders for influenza and pneumococcal immunizations, diabetes lab work, eye exam referrals, and diabetic foot screenings. Providers are presented with decision support for only those elements of diabetes bundle care that require complex medical decision making, including glycated hemoglobin (A1C), low-density lipoprotein cholesterol (LDL), and BP management. Patient report cards were generated during the office visit to be shared later with the patient. The individual metrics and all-or-none bundle results for each clinic team and for each individual provider were reported monthly. Each team had access to the individual reports and team reports of all other physicians/ teams within Geisinger.
Comparison Primary Care Population
Analysis of outcomes was based on claims data obtained from GHP. As previously noted, GHP members in the DS were compared with those in the NDS. The analysis was confined to GHP members because medical claims data on all eligible diabetes patients could be used to identify relevant documentation of micro- and macrovascular events. Analysis was limited to the period of services provided and paid by GHP from January 1, 2004, to December 31, 2008, and was confined to patients who were aged 40 years or older with a diabetes diagnosis. The 2 years of observations from 2004 to 2005 were used to establish baseline event rates in the two patient populations before the DS was implemented.
During the study period, individuals were included as diabetes cases if they met Healthcare Effectiveness Data and Information Set (HEDIS) criteria19 and had 4 or more encounters with a diabetes International Classification of Diseases, Ninth Revision (ICD-9) code on different dates during the study period. The earliest encounter with a diabetes ICD-9 code was taken as the diabetes baseline date. Patients had to have at least 180 days of follow-up after their diabetes baseline date to contribute to the analysis.
Outcomes
We identified all inpatient and outpatient claims during the 5-year study period related to the following ICD-9 codes: retinopathy (ICD- 9 codes 250.5x and 362.xx); amputation (ICD-9 codes 89.5x, 89.6x, 89.7x, and 99.7x); MI (ICD-9 code 410.xx); and stroke (ICD-9 codes 430, 431.xx-438.xx). The following numbers of cases were identified: 2518 retinopathy; 138 amputation; 3824 MI; and 2112 stroke. Incident microvascular or macrovascular events were identified as the earliest documentation of 1 of the above codes if there was also at least 1 year of follow-up prior to the first documentation of the claims event. This “clean-out period” restriction was imposed to eliminate prevalent events among those who became new members of GHP. In sensitivity analysis, we also used a 2-year clean-out period. The findings were essentially the same. Using the 1-year criteria, we identified 519 retinopathy, 17 amputation, 714 MI, and 445 stroke incident events.
Chronic kidney disease, 1 of 3 serious microvascular diseases associated with diabetes, was not evaluated as an outcome because it is not reliably reported in insurance claims data unless a patient has end-stage disease.
Statistical Analysis
Because patients were not randomized, we used a 2-step method to protect against inferential threats attributable to baseline differences in risk factors. First, logistic regression was used to calculate a propensity score20 for each eligible diabetes patient to balance disease burden for members of the DS and NDS groups at the beginning of intervention (January 1, 2006), which was denoted as the index date. If patients had pure postintervention exposure, then the index date was set to be the same as the baseline date. Claims data from the date of enrollment to the index date were used to derive the propensity score based on patient gender and age at index date, as well as claims documentation of comorbidities (mild liver disease, peripheral vascular disease, heart failure, cerebrovascular accident, pulmonary disease, severe liver disease, renal disease, malignant cancer, cardiovascular disease, and dialysis) up to the index date. The propensity score was used to match DS and to NDS diabetes patients (± 0.2 of SD of the propensity score, an optimal caliper usually used in propensity score—matching studies21) and to balance both groups on future risk of events of interest. Second, to account for differences in person-time of observation in the postsystem-of-care periods, we calculated the probability of being “exposed” to the post-system period. To this end, each patient’s age at diabetes baseline date, duration of person-time in the preintervention period, and comorbidities documented before the index dates were included in the conditional logistic regression model. Conditional logistic regression was used to control for matched pair variation. A score obtained for each subject represented the likelihood of postsystem-of-careexposure.
Person-time was excluded until a patient reached 40 years of age. Cumulative risk was estimated using the weighted Cox Proportional Hazards regression model, which combined a traditional Cox Proportional Hazard regression model with inverse probability weighting (IPW). The inverse of the previously described postsystem exposure score was used as a weight, along with age at the index date and gender. A patient was censored from observation if they discontinued as a GHP member, died as a GHP member, or incurred their first unique major microvascular or macrovascular event as previously described. The C statistic was used to measure discriminatory capability of the model and calculated as described by Pencina and colleague’s method.22 The cumulative hazard of each outcome was predicted by the model. Statistical analyses were performed using SAS version 9.2 (SAS Institute Inc, Cary, North Carolina) for the time-to-event analysis and relative risk comparison.
The number of patients needed to treat (NNT) was calculated based on risk reduction, which was estimated from the above Cox proportional hazard model; that is, the 3-year survival rate was estimated from the model for DS and NDS group, respectively, and the difference represented the 3-year risk reduction. The NNT is the inverse of the risk reduction.
RESULTS
Figure 1
Table 2
Table 3
The initial study cohort of 16,086 GHP members met the criteria for diabetes diagnosis. (). After applying exclusion criteria (<180-day follow-up, age <40, only preintervention exposure or prevalent outcome), the sample was reduced by 3421 of the 8355 patients in the DS group clinics and by 3634 of the 7734 in the NDS group clinics. After applying propensity score—matching criteria, 4095 DS subjects were matched to 4095 NDS subjects. Before matching (), DS group members were significantly older, had proportionately more of their person- time in the postintervention period, and had a longer follow-up period than NDS members. After matching, DS and NDS members still differed on duration of follow-up time in the postintervention period. The 2 groups were similar on the risk score derived at baseline and up to the time of the index date. The adjusted hazard ratio () for MI was 0.77 (95% CI, 0.65-0.90); for stroke, 0.79 (95% CI, 0.65-0.97); and for retinopathy, 0.81 (95% CI, 0.68- 0.97). The risk of the macrovascular and microvascular events were significantly lower among DS patients compared with NDS patients (Figure 2 includes cumulative hazard plots). These hazard plots show that the reduction in macrovascular outcomes (MI and stroke) is most pronounced after year 1 of the DS, and that the microvascular outcome retinopathy is evident after approximately 18 months. The risk of developing retinopathy, MI, and stroke among DS patients was significantly less than that of NDS patients in the 3-year follow-up period. The NNT to prevent 1 event over 3 years was 82 (95% CI, 37-133) for MI; 178 (95% CI, 57-681) for stroke; and 151 (95% CI, 47- 512) for retinopathy.
DISCUSSION
This analysis of claims data from a propensity-matched observational design shows a statistically significant lower risk of macrovascular and microvascular disease end points in the first 3 years of a diabetes system of care that included an all-or-none bundled measure compared with primary care without this intervention. The impact was substantial, with only 82 patients needed to treat to prevent 1 MI event, 178 to prevent 1 stroke, and 151 to prevent 1 case of retinopathy. Perhaps the most notable finding is the apparent early impact of the care model. The findings suggest an impact in the first 3 years with the possibility that a reduction in risk began to emerge after the first year. This finding is consistent with prior randomized controlled trials indicating that reduction in risk of cardiovascular outcomes can be achieved. 4-7,10 However, the early impact of an all-ornone diabetes system of care on microvascular and macrovascular conditions has not been previously described.
The finding of a reduction of MI, stroke, and retinopathy in only 3 years through a risk factor intervention is supported by prior studies on individual risk factors such as smoking cessation, BP control, and influenza immunization. 12,23-26 Multiple trials have also shown statistically significant improvements in microvascular and macrovascular outcomes in 3 to 5 years of follow-up in programs designed to reduce cardiovascular risk.4-7,10 This study showed improvement in a shorter period of followup. Observing statistically significant differences strongly depends on sample size, and showing statistically significant differences does not indicate when the benefits of an intervention first emerge. These previous trials were designed to show that the intervention had an effect, not to determine the earliest point at which the effect occurred. The current observational study included multiple simultaneous interventions which could have amplified the early benefits in risk reduction when compared with other studies using a single intervention.
A limitation of this study is that the DS group intervention did not occur in isolation.After the initiation of the DS, other performance improvement projects were initiated, including reporting of diabetic foot examination rates, diabetic eye examination rates, aspirin use, and angiotensin- converting enzyme inhibitor/angiotensin II receptor blocker use. However, it is unlikely that these other initiatives contributed to the early separation in risk observed in the DS clinics compared with the NDS clinics. The NDS sites also had other ongoing care improvements sponsored by the health plan. These included Web-based registry tools and outreach programs based on HEDIS measures for diabetes, which could have resulted in improved diabetes outcomes, but in a less substantial manner than in the DS sites. The DS intervention included many interventions, including physician and staff monetary incentives. These were used at the DS sites to focus attention and promote team attainment of goals. NDS sites also received a Physician Quality Summary bonus from GHP, but the monetary incentives were less than what was provided the DS sites and not related to an all-or-none improvement. The extent to which these varying monetary incentives contributed to the success of the intervention was not studied.
Finally, patients in the DS care arm were more likely to be cared for in practices that are a part of an integrated health system and in sites that have an EHR. While we used propensity score matching at the time of the index date to balance the “intervention” and control arms, there is the possibility that the separation of the time-toevent curves for micro- and macrovascular disease risk was explained by unmeasured confounding attributable to a host of factors that are simply correlated with the effectiveness of delivering the diabetes care.15,27
This diabetes system of care had multiple interventions, and the impact of each will require further research. Determining the best combination of bundled measures, work flow redesign, financial incentives, and the relative impact of each aspect of the system of care are ongoing.28,29 Analysis of the impact of the system of care on overall mortality and total cost of care is planned.
Prior evidence indicates that it is possible to improve process of care measures for diabetes in clinical practice, 30 and document small to modest improvements in glycemic control.31 There is a single report of reduced adverse events (amputations, retinopathy, and MI) using a diabetes disease management program.32 If substantiated, our findings that a DS reduces the microvascular and macrovascular complications of diabetes in only 3 years have potentially important implications for patients, providers, and payers. 33Author Affiliations: Geisinger Health System, Danville, PA (FJB, TRG, TA, DED, SBP, GDS); Sutter Health, Sacramento, CA (XY, WFS, JP).
Source of Funding: Geisinger Health Plan.
Author Disclosures: Drs Bloom and Graf report accepting fees from the Merck Speaker’s Bureau. Dr Davis reports employment with a group practice that operates as part of Geisinger Health System, from which data were used as part of this study. The other authors (XY, WFS, TA, SBP, JP, GDS) report no relationship or financial interest with any entity that would pose a conflict of interest with the subject matter of this article.
Authorship Information: Concept and design (FJB, XY, WFS, TA, SBP, GDS); acquisition of data (FJB, XY, TA, SBP, JP, GDS); analysis and interpretation of data (FJB, XY, WFS, TA, JP, GDS); drafting of the manuscript (FJB, WFS, TA, DED, JP, GDS); critical revision of the manuscript for important intellectual content (FJB, XY, WFS, TA, DED, JP, GDS); statistical analysis (XY); obtaining funding (DED); administrative, technical, or logistic support (FJB, TA, SBP, JP, GDS); supervision (FJB, WFS, JP, GDS).
Address correspondence to: Frederick J. Bloom Jr, 100 N. Academy Ave, Danville, PA 17822-3836. E-mail: fbloom@geisinger.edu.1. Health, United States, 2010: with special feature on death and dying. Report no. 2011-1232. National Center for Health Statistics: Hyattsville, MD; 2011.
2. The Diabetes Control and Complications Trial Research Group. The effect of intensive treatment of diabetes on the development and progression of long-term complications in insulin-dependent diabetes mellitus. N Engl J Med. 1993;329(14):977-986.
3. Stratton IM, Adler AI, Neil HA, et al. Association of glycaemia with macrovascular and microvascular complications of type 2 diabetes (UKPDS 35): Prospective observational study. BMJ. 2000;321(7258): 405-412.
4. Action to Control Cardiovascular Risk in Diabetes Study Group, Gerstein HC, Miller ME, et al. Effects of intensive glucose lowering in type 2 diabetes. N Engl J Med. 2008;358(24):2545-2559.
5. ADVANCE Collaborative Group, Patel A, MacMahon S, et al. Intensive blood glucose control and vascular outcomes in patients with type 2 diabetes. N Engl J Med. 2008;358(24):2560-2572.
6. Duckworth W, Abraira C, Moritz T, et al. Glucose control and vascular complications in veterans with type 2 diabetes. N Engl J Med. 2009;360(2):129-139.
7. Colhoun HM, Betteridge DJ, Durrington PN, et al. Primary prevention of cardiovascular disease with atorvastatin in type 2 diabetes in the collaborative atorvastatin diabetes study (CARDS): multicentre randomised placebo-controlled trial. Lancet. 2004;364(9435):685-696.
8. Collins R, Armitage J, Parish S, Sleigh P, Peto R, Heart Protection Study Collaborative Group. MRC/BHF heart protection study of cholesterol-lowering with simvastatin in 5963 people with diabetes: A randomised placebo-controlled trial. Lancet. 2003;361(9374):2005-2016.
9. Turnbull F, Neal B, Algert C, et al. Effects of different blood pressurelowering regimens on major cardiovascular events in individuals with and without diabetes mellitus: results of prospectively designed overviews of randomized trials. Arch Intern Med. 2005;165(12):1410-1419.
10. Gaede P, Lund-Andersen H, Parving HH, Pedersen O. Effect of a multifactorial intervention on mortality in type 2 diabetes. N Engl J Med. 2008;358(6):580-591.
11. Buse JB, Ginsberg HN, Bakris GL, et al. Primary prevention of cardiovascular diseases in people with diabetes mellitus: A scientific statement from the American Heart Association and the American Diabetes Association. Circulation. 2007;115(1):114-126.
12. Rea TD, Heckbert SR, Kaplan RC, Smith NL, Lemaitre RN, Psaty BM. Smoking status and risk for recurrent coronary events after myocardial infarction. Ann Intern Med. 2002;137(6):494-500.
13. American Diabetes Association. Standards of medical care in diabetes-- 2011. Diabetes Care. 2011;34(suppl 1):S11-S61.
14. Saaddine JB, Cadwell B, Gregg EW, et al. Improvements in diabetes processes of care and intermediate outcomes: United states, 1988-2002. Ann Intern Med. 2006;144(7):465-474.
15. Cebul RD, Love TE, Jain AK, Hebert CJ. Electronic health records and quality of diabetes care. N Engl J Med. 2011;365(9):825-833.
16. Weber V, Bloom F, Pierdon S, Wood C. Employing the electronic health record to improve diabetes care: a multifaceted intervention in an integrated delivery system. J Gen Intern Med. 2008;23(4):379-382.
17. American Diabetes Association. Standards of medical care in diabetes. Diabetes Care. 2005;28(Suppl 1):S4-S36.
18. Bloom FJ, Jr, Graf T, Anderer T, Stewart WF. Redesign of a diabetes system of care using an all-or-none diabetes bundle to build teamwork and improve intermediate outcomes. Diabetes Spectrum. 2010;23(3):165-169.
19. HEDIS 2009. Volume 2: Technical Specifications. Washington, DC: NCQA; 2008: 134-137.
20. Roosenbaum PR, Rubin DB. The central role of the propensity score in observational studies for causal effect. Biometrika. 1983;70(1):41-55.
21. Austin PC. Some methods of propensity-score matching had superior performance to others: results of an empirical investigation and Monte Carlo simulations. Biom J. 2009;51(1):171-184.
22. Pencina MJ, D’Agostino RB. Overall C as a measure of discrimination in survival analysis: model specific population value and confidence interval estimation. Stat Med. 2004;23(13):2109-2123.
23. Kawachi I, Colditz GA, Stampfer MJ, et al. Smoking cessation and decreased risk of stroke in women. JAMA. 1993;269(2):232-236.
24. Wolf PA, D’Agostino RB, Kannel WB, Bonita R, Belanger AJ. Cigarette smoking as a risk factor for stroke: the Framingham study. JAMA. 1988;259(7):1025-1029.
25. Lindholm LH, Ibsen H, Dahlof B, et al. Cardiovascular morbidity and mortality in patients with diabetes in the losartan intervention for endpoint reduction in hypertension study (LIFE): A randomised trial against atenolol. Lancet. 2002;359(9311):1004-1010.
26. Gurfinkel EP, Leon de la Fuente R, Mendiz O, Mautner B. Flu vaccination in acute coronary syndromes and planned percutaneous coronary interventions (FLUVACS) study. Eur Heart J. 2004;25(1):25-31.
27. Casalino LP. Which type of medical group provides higher-quality care? Ann Intern Med. 2006;145(11):860-861.
28. Shubrook JH,Jr, Snow RJ, McGill SL, Brannan GD. “All-or-none” (bundled) process and outcome indicators of diabetes care. Am J Manag Care. 2010;16(1):25-32.
29. Kelley E. All or none measurement: why we know so little about the comprehensiveness of care. Int J Qual Health Care. 2007;19(1):1-3.
30. Mangione CM, Gerzoff RB, Williamson DF, et al. The association between quality of care and the intensity of diabetes disease management programs. Ann Intern Med. 2006;145(2):107-116.
31. Shojania KG, Ranji SR, McDonald KM, et al. Effects of quality improvement strategies for type 2 diabetes on glycemic control: A metaregression analysis. JAMA. 2006;296(4):427-440. doi: 10.1001/jama .296.4.427.
32. Beaulieu N, Cutler DM, Ho K, et al. The business case for diabetes disease management for managed care organizations. Forum for Health Economics & Policy. 2006;9(1):1.
33. Paulus RA, Davis K, Steele GD. Continuous innovation in health care: implications of the Geisinger experience. Health Aff (Millwood). 2008;27(5):1235-1245.
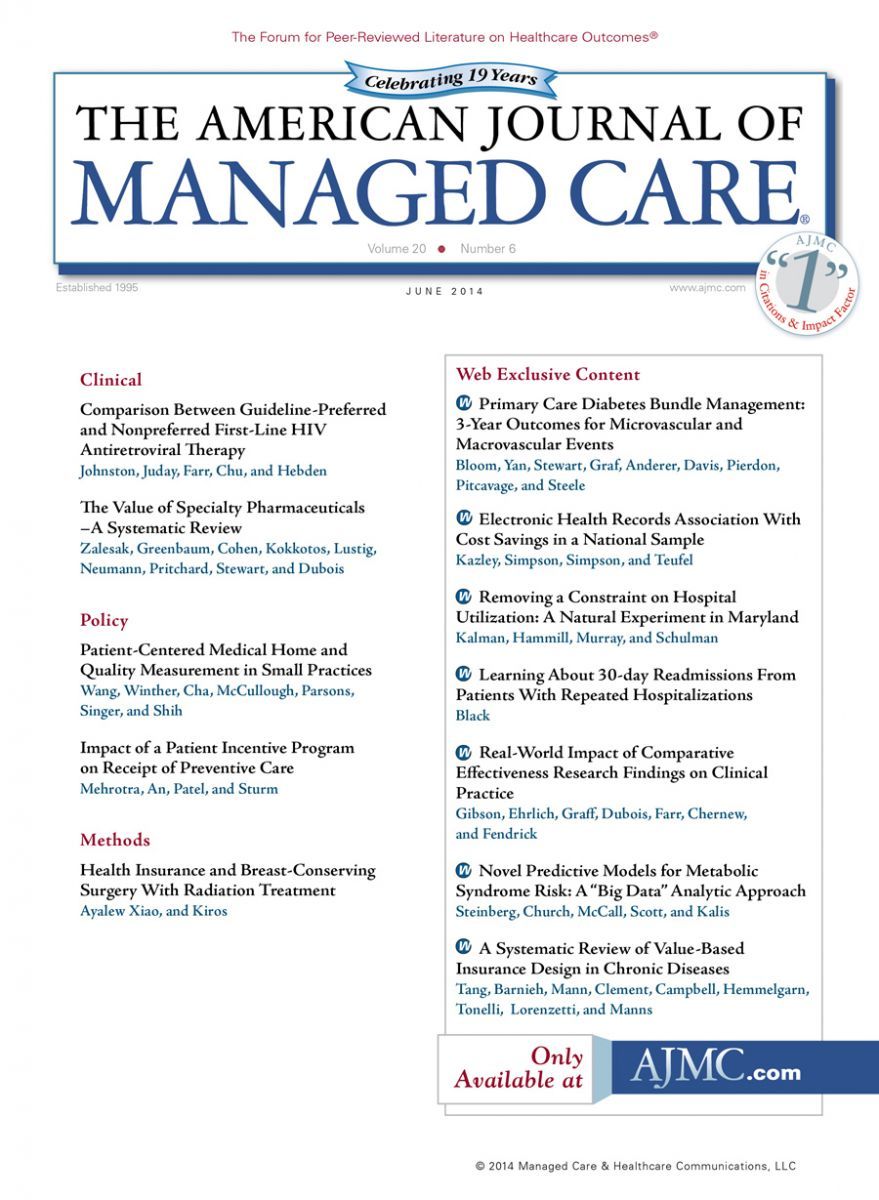