Publication
Article
Primary Care Visit Cadence and Hospital Admissions in High-Risk Patients
Author(s):
Patients with congestive heart failure and/or chronic obstructive pulmonary disease who had more quarterly primary care visits had lower rates of hospitalizations during the COVID-19 pandemic.
ABSTRACT
Objective: To determine whether high-cadence primary care visits are associated with reduced hospitalizations in high-risk patients with congestive heart failure (CHF) and/or chronic obstructive pulmonary disease (COPD).
Study Design: Retrospective cohort study using Medicare Advantage claims.
Methods: High-risk patients from 14 primary care clinics were encouraged to receive quarterly visits through a quality improvement program. Cadence was determined by the number of quarterly visits in a 12-month period. We compared the hospitalizations in 1342 high- and low-cadence patients during the base year and following year adjusting for patient characteristics and health risks.
Results: Patients with CHF and/or COPD had a mean Hierarchical Condition Category (HCC) score of 1.98 during the baseline year. High-cadence patients at the baseline year (n = 881) had comparable admissions per thousand (ADK) in both years (first year: 199; 95% CI, 174-227; second year: 196; 95% CI, 171-223; P = .88), and low-cadence patients (n = 461) had elevated ADK in the second year (first year: 62; 95% CI, 44-88; second year: 117; 95% CI, 90-149; P < .05). ADK also increased across all HCC quartiles among the low-cadence patients. In contrast, year-over-year ADK declined or was flat among the high-cadence population for the bottom 3 HCC quartiles. Adjusted regression analyses confirmed the suggestive evidence of reduced ADK in baseline high-cadence patients (coefficient, –0.63; 95% CI, –1.22 to –0.05; P = .033).
Conclusions: High cadence of primary care visits was associated with a significant reduction in hospitalizations among high-risk patients. The impact of visit cadence on patient outcomes may need to be tested in randomized interventions.
Am J Manag Care. 2024;30(6):263-269. https://doi.org/10.37765/ajmc.2024.89509
Takeaway Points
This quality improvement study of 1342 patients with congestive heart failure and/or chronic obstructive pulmonary disease at 14 primary care clinics found that those with more quarterly primary care visits had lower rates of hospitalizations during the COVID-19 pandemic.
- High-cadence primary care visits may allow an opportunity to reduce hospitalization in high-risk patients.
- Primary care providers need to improve the regularity of visits among high-risk patients.
- Future research is needed to investigate patient engagement in visit cadence programs, the impact of visit cadence on different patient outcomes in different payer arrangements, and the potential long-term effects of the quality improvement program.
Regularity of visits is a core part of longitudinal primary care.1-4 It emphasizes the need for “regular, proactive, and planned visits”4 to ensure disease management, continuity of care, and successful care coordination.4-6 However, there is a paucity of research to guide a primary care provider (PCP) on the optimal interval of routine visits. Irregular visits may allow unaddressed health issues to worsen, particularly among disadvantaged patients with complex needs, resulting in preventable hospitalizations. A recent study of traditional Medicare beneficiaries demonstrated that average-risk patients, characterized by a mean Hierarchical Condition Category (HCC) score of 1.14, had fewer emergency visits and hospitalizations and lower health care costs when they maintained regular visits with PCPs.3 Frequent primary care visits in the US Department of Veterans Affairs Health Care System were associated with statin adherence.7 However, no previous study has examined whether high-cadence primary care visits reduce hospitalizations in high-risk patients with Medicare Advantage.
The decision of visit cadence is influenced by various factors including the patient’s preferences, future appointment availability, socioeconomic status, and health insurance payment design.1 Visit cadence is a fundamental component of value-based primary care within capitation reimbursement models.4 An increasing share of payers has shifted to paying for value since the passage of the Affordable Care Act,8 thus rewarding PCPs for caring for complex patients and reducing the effects of chronic conditions.9 Value-based PCPs often determine the regularity of visits based on patients’ health risks.4 A patient engagement program (Village Medical Stay Well) was implemented to encourage quarterly primary care visits among patients with congestive heart failure (CHF) and/or chronic obstructive pulmonary disease (COPD). However, limited studies have examined the results of visit cadence programs in value-based primary care.3
Stay Well is a patient outreach and engagement initiative launched in 2019 to promote at least quarterly visits to PCPs for patients with high disease burden, specifically targeting those with chronic conditions. The program was initially focused on patients with CHF and/or COPD but has since expanded to encompass a broader range of conditions. The program’s workflow utilizes electronic health records (EHRs) to preschedule appointments for all eligible patients. Following each visit, patients are scheduled for their subsequent quarterly follow-up. The EHR system also serves to monitor and manage the visitation schedule for these patients. In addition, regular operational review meetings are held to optimize scheduling and to ensure the program goals are met.
Although the COVID-19 pandemic led to interruptions in routine primary care,10 the patient engagement program persevered and was consistently carried out throughout 2020 and 2021. This study described the visit cadence of primary care among patients with CHF and/or COPD and evaluated the influence of visit cadence on hospitalizations in patients enrolled in the program using a multivariate regression analysis adjusting for patient characteristics.11,12 These patients were enrolled in Medicare Advantage plans, receiving their primary care services from a network of clinics in Houston, Texas. We also conducted a similar secondary analysis in usual care patients with lower health risk scores.
METHODS
Design, Setting, and Data Sources
The study cohort for this retrospective analysis consisted of Medicare Advantage patients from 14 primary care clinics in Houston. We used their medical records and claims from July 2019 to June 2021. We used data from the first year (July 2019 through June 2020) as a baseline period. The last quarter of the first year coincided with the lockdown period at the beginning of the COVID-19 pandemic. Another 4981 usual care patients were included for the secondary analysis. Limitations with the retrospective cohort study design are described in the Discussion.
Study Population
Our study began by identifying the Medicare Advantage population in Houston that was attributed to Village Medical as of July 2019, marking the start of our study period (N = 17,416). Patients were included if they were continuously enrolled in Medicare Advantage and attributed to Village Medical during the 24 months of the study period. Figure 1 shows the sample exclusion criteria. For example, we excluded deceased patients and patients hospitalized due to COVID-19. Patients with specific high-cost procedures or comorbid conditions (joint replacement, trauma, cancer, end-stage kidney disease) were also excluded. The final study cohort (n = 6323) comprised 1342 high-risk patients from the quality improvement program and 4981 usual care patients. High-risk patients enrolled in Stay Well during the study period all received a diagnosis of CHF and/or COPD before July 2019. No patients who received a new diagnosis of CHF and/or COPD during the study were included. Usual care patients without CHF or COPD were included for the secondary analysis.
Visit Cadence and Patient Groups
The value-based primary care model involved completing a visit every 90 days for the patients with CHF and/or COPD in the program. To determine adherence to the planned visit cadence, we measured the unique number of quarters a patient was seen in the baseline year using the following segments: July 2019 to September 2019, October 2019 to December 2019, January 2020 to March 2020, and April 2020 to June 2020. All in-person and virtual primary care encounters were included (see eAppendix Table 1 [eAppendix available at ajmc.com]), including annual wellness visits, welcome to Medicare visits, office visits, home visits, and physicals. Visits billed as transitional care management after acute hospitalization were not included because these visits are more reactive than proactive primary care and including them would result in statistical bias.
Patients in the program were considered to be in the high-cadence group if they were seen in at least 3 quarters in view of delayed visits, scheduling conflicts, and the unprecedented challenge caused by the COVID-19 pandemic. We defined low-cadence patients as those who had 2 or fewer quarterly primary care visits. We compared hospitalizations between high-cadence and low-cadence patients during the base year and the following year, adjusting for patient characteristics and risks. For the secondary analysis of usual care patients, we created 2 patient groups based on their primary care visit cadence: patients receiving primary care in 3 or 4 quarters in the baseline year and patients receiving primary care in fewer than 3 quarters in the baseline year.
Hospitalization and Covariates
Inpatient claims were used to determine hospitalizations during the baseline and following year. Hospitalizations were counted as unique acute inpatient stays, possibly across multiple facilities, with transfers not constituting separate admissions. The primary outcome was admissions per thousand beneficiaries (ADK), which was calculated by dividing the total number of admissions by the total number of beneficiaries and then multiplying the result by 1000. In the subsequent text, ADK and hospitalization rates are used interchangeably. Similarly, the terms high-risk patients and patients with CHF and/or COPD are also used interchangeably. Patient demographic characteristics included age, sex, and race, and health risk was measured by HCC score. Demographic data were obtained in the baseline year and were not reassessed in the second year. Race and ethnicity were self-reported within the EHR. HCC scores were determined at the start of the baseline year without applying the normalization factor.
Statistical Analysis
Baseline health risk and health care utilization confound the relationship between visit cadence and hospitalization. We performed a retrospective analysis evaluating the influence of high-cadence primary care visits in the baseline year on hospitalizations in the following year among patients with CHF and/or COPD. This approach accounts for confounders “that change differently over time in the treated and comparison group or have a time-varying effect on the outcome,” according to Zeldow and Hatfield.11-13 For example, the hospital admission rates were significantly reduced regardless of patient risk level during the COVID-19 lockdown in 2020. This exogenous effect likely had a similar impact on high-cadence and low-cadence patients.
Considering hospital admission in a given patient-year as a binary outcome, we used a logistic regression model with covariates, including a binary indicator for the year (0 and 1 for the baseline and following year, respectively), an indicator for cadence with the program (0 and 1 for low-cadence and high-cadence patients, respectively), individual-level characteristics (age, gender, race, HCC score), and an interaction term between the year and cadence group. The regression coefficient of the interaction term represents the estimated association between high-cadence primary care visits in the baseline year and hospitalizations in the following year. The dependent variable was a patient’s hospitalization status in the following year.
We performed a similar retrospective analysis in usual care patients. All statistical analyses used R software version 3.3.2 (R Project for Statistical Computing) or Python version 3.10 (Python Software Foundation) with the Python statistical analysis packages statsmodels 0.13.2 and SciPy 1.9.0. Two-sided P values less than .05 indicated significance.
RESULTS
Patient Characteristics and Primary Care Visit Cadence
The study sample of the primary analyses included 1342 unique patients from the Village Medical Stay Well program.The mean (SD) age was 75.8 (8.4) years; 692 (51.6%) were female; 1013 (75.5%) were White; and 605 (45.1%) had CHF, 939 (70.0%) had COPD, and 202 (15.1%) had both CHF and COPD (Table 1). The mean HCC score was 1.98 during the baseline year. Despite the COVID-19 interruptions, approximately 66% of patients received primary care at a high cadence (Table 1). Similar numbers of patients were seen by PCPs in 3 and 4 quarters (436 and 445, respectively) during the baseline year. Of the remaining low-cadence patients, 311 (23.2%) were seen in 2 quarters. Only 11.1% of patients were seen in 1 quarter of the year or not seen at all. On average, patients with CHF and/or COPD in the program were seen in 2.9 quarters during the baseline year. The primary care visit frequency and HCC score were not statistically correlated with patient demographics (see eAppendix Table 2), except for an expected positive correlation between age and HCC score. Usual care patients (n = 4981) in the secondary analysis had a mean HCC score of 0.99 and were seen on average in 2.4 quarters, with approximately 48% seen in at least 3 quarters.
Hospitalization Rates
Figure 2 shows that the ADK in low-cadence high-risk patients (n = 461) increased from 62 (Wilson 95% CI, 44-88) to 117 in the following year (95% CI, 90-149; paired t test, t = 2.36; P < .05). In contrast, high-cadence patients (n = 881) had comparable admission rates in both years (first year: 199; 95% CI, 174-227; second year: 196; 95% CI, 171-223; P = .88). A similar pattern was observed among usual care patients. The ADK in patients receiving primary care in fewer than 3 quarters increased from 37 in the first year (95% CI, 30-45) to 50 in the second year (95% CI, 42-59; P < .05). Patients receiving primary care in 3 or 4 quarters in the baseline year had similar ADK in both years (first year: 89; 95% CI, 79-102; second year: 76; 95% CI, 66-87; P = .12). eAppendix Figure 1 shows the correlation between hospitalization rates in both years and the number of quarters in which patients were seen by PCPs during the baseline year.
Moreover, hospitalization rates increased across all HCC quartiles among the low-cadence patients with COPD and/or CHF (Figure 3). In contrast, year-over-year ADK declined or was flat among the high-cadence population in the bottom 3 HCC quartiles. For example, low-cadence patients in the third HCC quartile experienced a 22% increase in hospitalization rates, whereas the ADK in high-cadence patients in the third quartile remained the same in the following year. A similar pattern was also observed among the usual care patients.
Regression Estimates
Within the Stay Well program, high-risk patients who had more frequent primary care visits (quantified by the number of quarters seen by PCPs) demonstrated higher baseline ADK (eAppendix Figure 1) and higher baseline HCC risk scores (eAppendix Figure 2) compared with those with less frequent visits. Table 2 presents regression coefficients for whether high-cadence patients experienced a reduction in hospitalization probabilities. The regression results suggest a decrease in admission log odds of –0.63 (95% CI, –1.22 to –0.049; P = .033). This decrease indicates a 47% relative reduction in the odds of hospitalization from the first to the second year for patients in the high-cadence group compared with the change in the low-cadence group.
Upon repeating the regression analysis in the usual care population, we observed a similar negative coefficient of –0.56 (95% CI, –0.92 to –0.20; P = .002). Usual care patients receiving primary care in 3 or 4 quarters had 43% lower odds of hospitalization in the second year than patients receiving primary care in fewer than 3 quarters.
DISCUSSION
Despite the disruptions to chronic care during the pandemic, the Stay Well program managed to help 66% of patients with CHF and/or COPD receive primary care services at high cadence. Although regular visits are essential in value-based primary care, few studies have examined the value of visit cadence in Medicare Advantage or capitation payment models in traditional Medicare. Using a large cohort of high-risk patients with Medicare Advantage, we examined whether high-cadence primary care visits were associated with reduced hospitalizations during the COVID-19 pandemic. The odds of hospitalization in the second year among high-risk patients receiving primary care in 3 or 4 quarters of the first year were 47% lower than among patients receiving primary care in fewer than 3 quarters. During the 2-year study, an increase in hospitalizations from the first to the second year was noted in the group with a lower visit cadence in the initial year. This pattern was not evident among those with a higher visit cadence. These findings are consistent with those of an observational evaluation of primary care visit regularity and health care utilization in traditional Medicare beneficiaries receiving care at federally qualified health centers.3 In contrast, a Humana study found that PCP encounter regularity was not associated with inpatient admissions in patients with newly diagnosed type 2 diabetes.14 Future experimental studies are needed to investigate the effect of the frequency and regularity of patient visits on health outcomes. An advanced computational model could be employed to generate tailored visit frequency recommendations based on each patient’s risk profile and preferences.1,4
Our primary explanation of the findings from this study is that high-cadence primary care visits reflect a higher quality of care. In value-based primary care, patients are often assigned to risk tiers. Complex patients are proactively scheduled to see PCPs more often than low-risk patients. In this quality improvement program, COPD and CHF diagnoses indicate the need for a quarterly visit cadence for those primary care patients. Regular visits build a long-term patient-provider relationship that creates a “nonjudgmental emotional bond,” according to Scott et al.15 The bond ensures that the care team effectively diagnoses symptoms, manages conditions, sets health goals, and addresses any specific wellness concerns.16-18 Regular visits also enable patient interaction with the interprofessional primary care team.19 The planned visits emphasize disease management, care coordination, and continuity of care. For example, the management of COPD in primary care focuses on function improvement and symptom control through lifestyle changes, medication adjustment and adherence, preventive services, and care coordination.20-22 Prescribed PCP visits offer the opportunity to check patients’ lung function and symptoms, improve their self-care skills, and modify their treatment plans.
The variation in hospitalization rates in relation to primary care visit cadence may also be influenced by several interconnected factors. Changes in health status likely play a significant role, with those attending fewer visits possibly experiencing health deterioration leading to hospitalizations. Selection bias and inherently different characteristics between patients with varying levels of health care engagement, such as socioeconomic status and health literacy, could also skew results. Additionally, the quality of care, individual provider effectiveness, and hospital-level factors might affect outcomes.
Primary care providers without visit cadence planning programs may need to improve the regularity of visits among high-risk patients. Value-based care providers can determine visit frequency and regularity based on clinical judgment or by using machine-learning algorithms.3,4 The adherence to and impact of prescribed visit cadence will need to be monitored. The traditional fee-for-service payment model does not have quality incentives that align with the primary care visit regularity program. This partly explains the national decline in primary care visits “at a time when we may need primary care more than ever,” according to Ganguli et al.23 In contrast, value-based delivery models strive to provide the proper care at the right time for patients.24 Christakis suggested that “it is time to declare continuity of care an outcome.”25 Financial incentive from payers is needed to build long-term relationships between chronically ill patients and interprofessional primary care teams.26
Limitations
This study had several limitations. First, it shared the limits of the retrospective regression research design. We could not analyze hospitalization trends with additional years of data before the baseline year.11,13 We did not know how many hospitalizations would have happened in high-cadence patients in the second year if they had not received quarterly primary care. Although we anticipate that patients would exhibit similar avoidance of hospitals during the COVID-19 lockdown, the impact of the pandemic on health care–seeking behavior may vary by patient subgroups.27 Second, the control group (low-cadence patients) was imperfect because their baseline risk scores and hospitalization rates were significantly lower than those of high-cadence patients. However, the similar findings from both the quality improvement program and usual care patients made it seem less likely that unadjusted confounding accounts for all or most of the observed effects. Third, this study used only data from Medicare Advantage patients, so it is not clear how the findings would apply to other patient populations. The study did not account for visit cadence before or after the baseline year, focusing solely on the first year’s cadence and its impact on hospitalization changes over 2 years. Acknowledging this as a limitation, we aim to incorporate a broader data scope and more comprehensive analysis in future research. The study also did not examine the influence of subspecialty care on outcomes. Not tracking changes in HCC scores and visit regularity from year 1 to year 2 is another limitation of the study. Lastly, the sample size constrained our ability to examine effects at the clinic level.
In addition to randomized controlled interventions, future research needs to (1) investigate the reasons why 11% of high-risk patients were seen in only 1 quarter of the year or not seen at all, (2) examine whether visit cadence is associated with other patient outcomes14 in different payer arrangements, (3) quantify the potential greater long-term effects of the quality improvement program, and (4) determine the dose-response relationship between visit cadence and health care outcomes stratified by patient risk levels. For example, frail and/or homebound patients with an HCC score of 3 or greater may need monthly or bimonthly home-based primary care.28,29
CONCLUSIONS
This study’s findings suggest the importance of visit cadence as a fundamental element of value-based primary care models. They suggest a possible association between frequent primary care visits and a reduction in hospitalizations among high-risk patients. The continuity and regularity of care could be considered as a potential quality metric in value-based primary care.26 Primary care providers could potentially utilize machine-learning algorithms or rely on clinical judgment to determine visit cadence. The value-based care model of visit cadence planning also requires a focus on patient engagement and adherence. Strategies and tools need to be developed and implemented to achieve higher levels of patient engagement to increase access to regular primary care and improve patient outcomes.
Acknowledgments
Adam Matsil, MS, and Daniel Shenfeld, PhD, contributed equally to this work and are listed as co–first authors.
Author Affiliations: VillageMD (AM, CF, JC), Chicago, IL; Manganese Health Data Solutions (DS), Cambridge, MA; VillageMD Research Institute (AY), Chicago, IL; FastHSR (AY), Glen Allen, VA.
Source of Funding: None.
Author Disclosures: The authors report no relationship or financial interest with any entity that would pose a conflict of interest with the subject matter of this article.
Authorship Information: Concept and design (DS, CF, JC); acquisition of data (AM, JC); analysis and interpretation of data (AM, DS, AY, JC); drafting of the manuscript (AM, DS, AY, JC); critical revision of the manuscript for important intellectual content (AM, DS, CF, AY, JC); statistical analysis (AM, DS); provision of patients or study materials (CF, JC); administrative, technical, or logistic support (CF, AY, JC); and supervision (CF, JC).
Address Correspondence to: Aaron Yao, PhD, VillageMD Research Institute, 1 Diamond Hill Rd, Berkeley Heights, NJ 07922. Email: ayao@villagemd.com.
REFERENCES
1. Fihn SD, McDonell MB, Vermes D, et al. A computerized intervention to improve timing of outpatient follow-up : a multicenter randomized trial in patients treated with warfarin. National Consortium of Anticoagulation Clinics. J Gen Intern Med. 1994;9(3):131-139. doi:10.1007/BF02600026
2. Schwartz LM, Woloshin S, Wasson JH, Renfrew RA, Welch HG. Setting the revisit interval in primary care. J Gen Intern Med. 1999;14(4):230-235. doi:10.1046/j.1525-1497.1999.00322.x
3. Rose AJ, Timbie JW, Setodji C, Friedberg MW, Malsberger R, Kahn KL. Primary care visit regularity and patient outcomes: an observational study. J Gen Intern Med. 2019;34(1):82-89. doi:10.1007/s11606-018-4718-x
4. Bhatt S, Majerczyk N, Rose J, et al. Primary care visit cadence based on risk: a comparison of machine learning and provider judgment. NEJM Catal Innov Care Deliv. 2022;3(4). doi:10.1056/CAT.21.0322
5. Bodenheimer T, Wagner EH, Grumbach K. Improving primary care for patients with chronic illness. JAMA. 2002;288(14):1775-1779. doi:10.1001/jama.288.14.1775
6. Wagner EH, Austin BT, Von Korff M. Organizing care for patients with chronic illness. Milbank Q. 1996;74(4):511-544.
7. Ahmed ST, Mahtta D, Rehman H, et al. Association between frequency of primary care provider visits and evidence-based statin prescribing and statin adherence: findings from the Veterans Affairs system. Am Heart J. 2020;221:9-18. doi:10.1016/j.ahj.2019.11.019
8. Werner RM, Emanuel EJ, Pham HH, Navathe AS. The future of value-based payment: a road map to 2030. Leonard Davis Institute of Health Economics white paper. February 18, 2021. Accessed November 18, 2022. https://repository.upenn.edu/ldi_issuebriefs/149
9. What is value-based healthcare? NEJM Catalyst. January 1, 2017. Accessed November 18, 2022.
https://catalyst.nejm.org/doi/full/10.1056/CAT.17.0558
10. Matenge S, Sturgiss E, Desborough J, Hall Dykgraaf S, Dut G, Kidd M. Ensuring the continuation of routine primary care during the COVID-19 pandemic: a review of the international literature. Fam Pract. 2022;39(4):747-761. doi:10.1093/fampra/cmab115
11. Lissenden B, Yao NA. Affordable Care Act changes to Medicare led to increased diagnoses of early-stage colorectal cancer among seniors. Health Aff (Millwood). 2017;36(1):101-107. doi:10.1377/hlthaff.2016.0607
12. Sabik LM, Eom KY, Dahman B, et al. The impact of Massachusetts health reform on colorectal and breast cancer stage at diagnosis. Med Care. 2020;58(2):183-191. doi:10.1097/MLR.0000000000001241
13. Zeldow B, Hatfield LA. Confounding and regression adjustment in difference-in-differences studies. Health Serv Res. 2021;56(5):932-941. doi:10.1111/1475-6773.13666
14. Dobbins JM, Elliott SW, Cordier T, et al. Primary care provider encounter cadence and HbA1c control in older patients with diabetes. Am J Prev Med. 2019;57(4):e95-e101. doi:10.1016/j.amepre.2019.04.018
15. Scott JG, Cohen D, DiCicco-Bloom B, Miller WL, Stange KC, Crabtree BF. Understanding healing relationships in primary care. Ann Fam Med. 2008;6(4):315-322. doi:10.1370/afm.860
16. Weiss LJ, Blustein J. Faithful patients: the effect of long-term physician-patient relationships on the costs and use of health care by older Americans. Am J Public Health. 1996;86(12):1742-1747. doi:10.2105/AJPH.86.12.1742
17. Saultz JW, Lochner J. Interpersonal continuity of care and care outcomes: a critical review. Ann Fam Med. 2005;3(2):159-166. doi:10.1370/afm.285
18. van Walraven C, Oake N, Jennings A, Forster AJ. The association between continuity of care and outcomes: a systematic and critical review. J Eval Clin Pract. 2010;16(5):947-956. doi:10.1111/j.1365-2753.2009.01235.x
19. McCutcheon LRM, Haines ST, Valaitis R, et al. Impact of interprofessional primary care practice on patient outcomes: a scoping review. SAGE Open. 2020;10(2). doi:10.1177/2158244020935899
20. Hussey PS, Schneider EC, Rudin RS, Fox DS, Lai J, Pollack CE. Continuity and the costs of care for chronic disease. JAMA Intern Med. 2014;174(5):742-748. doi:10.1001/jamainternmed.2014.245
21. de Jong C, van Boven JFM, de Boer MR, Kocks JWH, Berger MY, van der Molen T. Improved health status of severe COPD patients after being included in an integrated primary care service: a prospective cohort study. Eur J Gen Pract. 2022;28(1):66-74. doi:10.1080/13814788.2022.2059070
22. Park HJ, Byun MK, Kim T, et al. Frequent outpatient visits prevent exacerbation of chronic obstructive pulmonary disease. Sci Rep. 2020;10(1):6049. doi:10.1038/s41598-020-63064-x
23. Ganguli I, Lee TH, Mehrotra A. Evidence and implications behind a national decline in primary care visits. J Gen Intern Med. 2019;34(10):2260-2263. doi:10.1007/s11606-019-05104-5
24. Ganguli I, Wasfy JH, Ferris TG. What is the right number of clinic appointments?: visit frequency and the accountable care organization. JAMA. 2015;313(19):1905-1906. doi:10.1001/jama.2015.3356
25. Christakis DA. Continuity of care: process or outcome? Ann Fam Med. 2003;1(3):131-133. doi:10.1370/afm.86
26. Yang Z, Ganguli I, Davis C, et al. Physician- versus practice-level primary care continuity and association with outcomes in Medicare beneficiaries. Health Serv Res. 2022;57(4):914-929. doi:10.1111/1475-6773.13999
27. Grubenhoff JA, Carubia BA. Understanding regional mental health care-seeking behavior during the COVID-19 pandemic—an important aspect of addressing resource needs. JAMA Netw Open. 2022;5(7):e2220560. doi:10.1001/jamanetworkopen.2022.20560
28. Yao N, Mutter JB, Berry JD, Yamanaka T, Mohess DT, Cornwell T. In traditional Medicare, modest growth in the home care workforce largely driven by nurse practitioners. Health Aff (Millwood). 2021;40(3):478-486. doi:10.1377/hlthaff.2020.00671
29. Yao NA, Ritchie C, Cornwell T, Leff B. Use of home-based medical care and disparities. J Am Geriatr Soc. 2018;66(9):1716-1720. doi:10.1111/jgs.15444
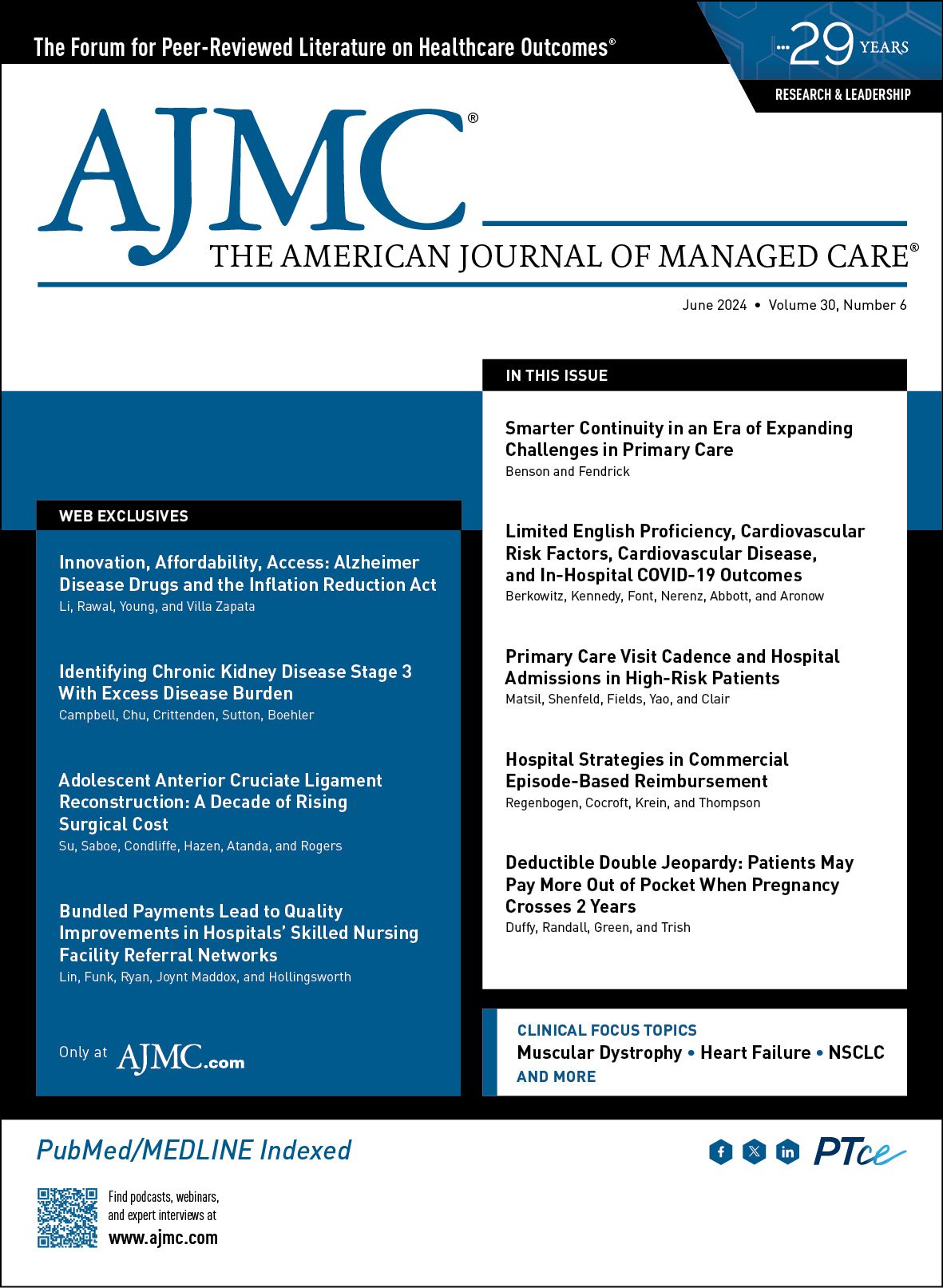